Companies Must Assess Threats to AI & ML Systems in 2022: Microsoft
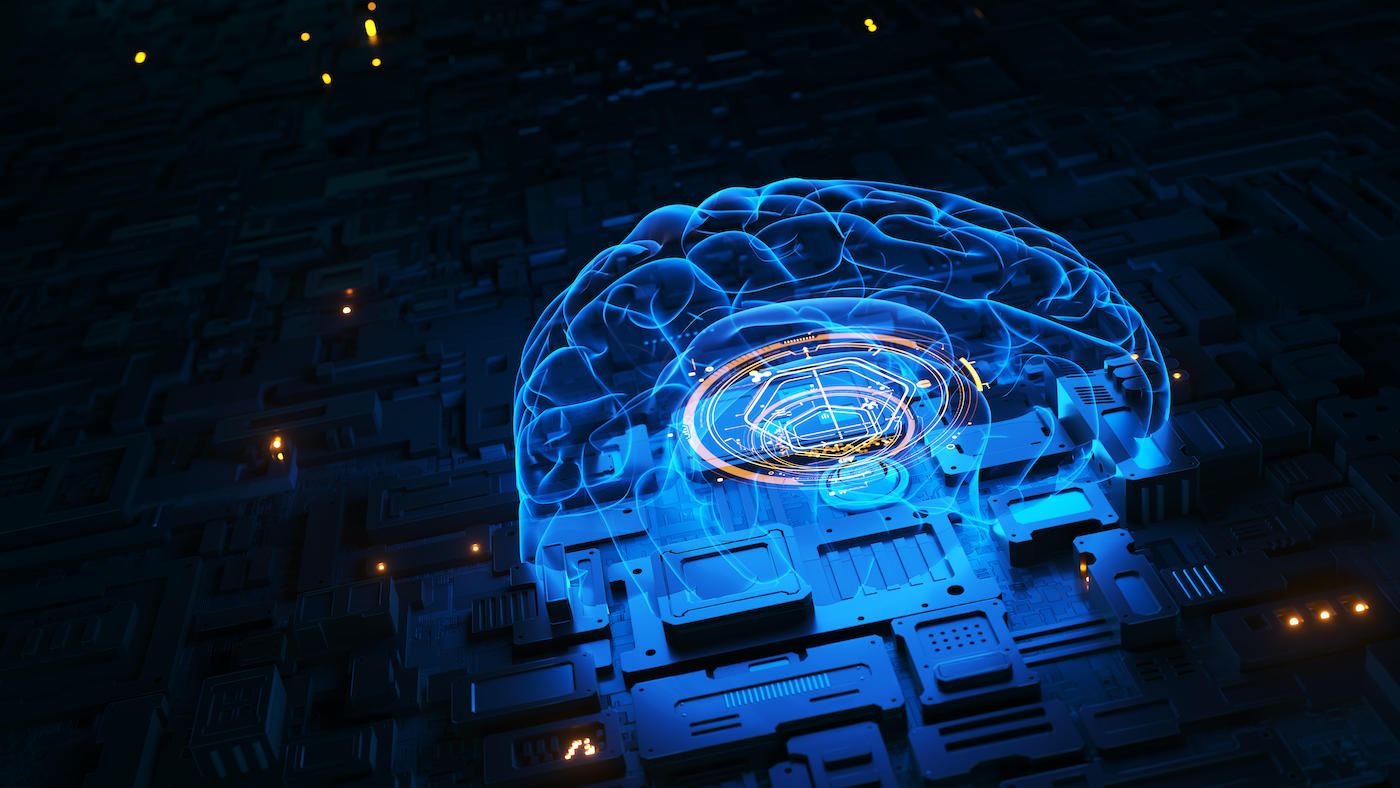
With the number of attacks on artificial intelligence (AI) and machine-learning (ML) systems rising, organizations must consider threats to their tools, systems, and pipelines as part of their security model and take steps to evaluate their risk.
Last week, Microsoft’s ML team published a framework that explains how organizations can gather information on their use of AI, analyze the current state of their security, and create ways of tracking progress. The report, “AI Security Risk Assessment,” argues that companies cannot, and need not, create a separate process for evaluating the security of AI and ML systems, but they should incorporate AI and ML considerations into current security processes.
Because many users of ML systems are not ML experts, the team focused on providing practical advice and tools, says Ram Shankar Siva Kumar, a “data cowboy” at Microsoft.
“These stakeholders cannot be expected to get a Ph.D. in machine learning to start securing machine learning systems,” he says. “We emphasize … crunchy, practical tools and frameworks … [and] contextualize securing AI systems in a language stakeholders already speak instead of asking them to learn an entirely new lexicon.”
This report is Microsoft’s latest effort to tackle what it sees as a growing gap between the security and popularity of AI systems. In addition to the report, Microsoft last week updated its Counterfit tool, an open source project that aims to automate the assessment of ML systems’ security. In July, the company launched the Machine Learning Security Evasion Competition, which allows researchers to test attacks against a variety of realistic systems and rewards those who can successfully evade security-focused ML systems, such as anti-phishing and anti-malware scanners.
While Microsoft has documented attacks against AI systems, such as the subversion of its chatbot Tay by a sustained online mob of miscreants, the company’s research found the vast majority of organizations did not have a workable security process to protect their systems.
“[W]ith the proliferation of AI systems comes the increased risk that the machine learning powering these systems can be manipulated to achieve an adversary’s goals,” the company said at the time. “While the risks are inherent in all deployed machine learning models, the threat is especially explicit in cybersecurity, where machine learning models are increasingly relied on to detect threat actors’ tools and behaviors.”
Companies still do not consider adversarial attacks on ML and AI systems a current threat but more of a future worry. In a March 2021 paper, Microsoft found only three of 28 companies interviewed had taken steps to secure their ML systems. Yet many continued to worry about future attacks on ML systems, such as one financial technology firm that feared an attack could skew its machine-generated financial recommendations.
“Most organizations are worried about their data being poisoned or corrupted by an adversary,” says Kumar. “Corrupting the data can cause downstream effects and disrupt systems, irrespective of the complexity of the underlying algorithm that is used.”
Other top concerns included attack techniques for learning the details of an ML model by observing the system at work, as well as attacks that extract sensitive data from the system, the survey found.
Microsoft’s report broke down the areas of AI systems into seven technical controls, such as model training and incident management, and a single administrative control, machine learning security policies. The technical control of data collection, for example, focused on requiring models to only use trusted sources of data from training and operations.
Most models today use untrusted data, which is a threat, the company explained.
“Data is collected from untrusted sources that could contain sensitive personal data, other undesirable data that could affect the performance of a model or presents compliance risks to the organization,” Microsoft listed among the threats in the report. “Data is stored insecurely and can be tampered with or altered by unauthorized parties or systems. Data is not correctly classified, leading to the disclosure of confidential information or sensitive personal data.”
The paper and automation tools are Microsoft’s latest efforts to create a formal way of defining AI threats and defenses against those threats. In February, the company urged organizations to think of ways to attack their AI systems as an exercise in creating defenses. Last year, Microsoft joined with government contractor MITRE and other organizations to create a classification of attacks, the Adversarial ML Threat Matrix.
Read More HERE