Academics Devise Cyber Intrusion Detection System For Unmanned Robots
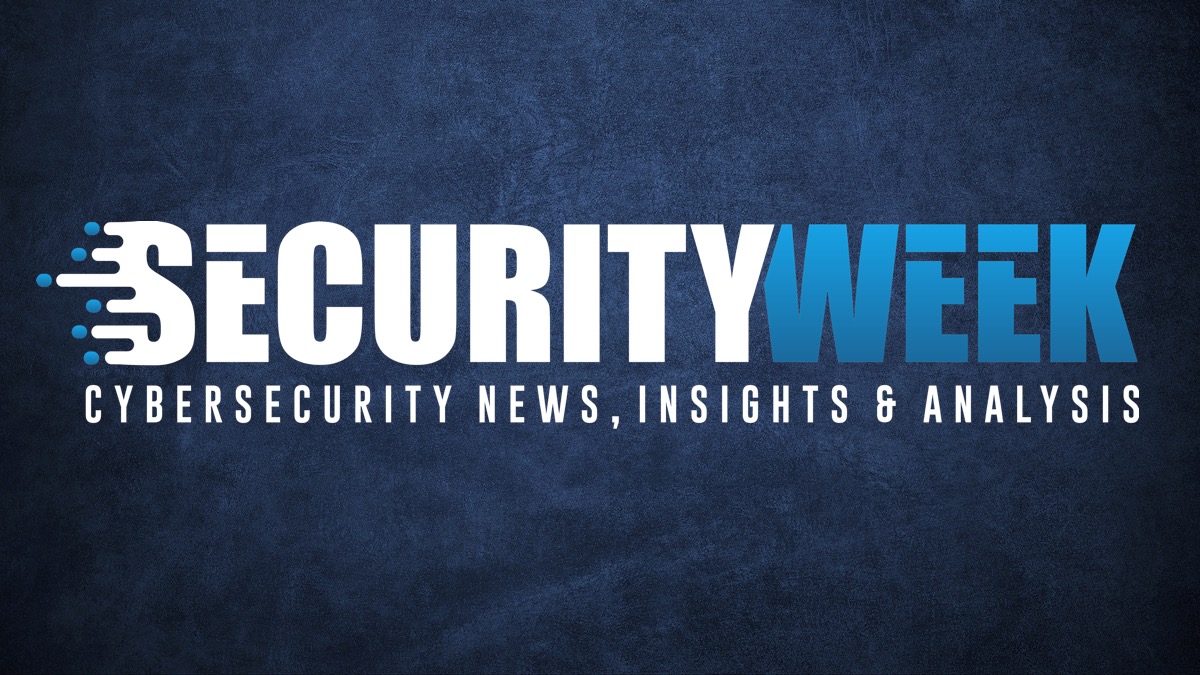
Two Australian academic researchers have devised a new cyber intrusion detection system that relies on AI to help unmanned military robots identify man-in-the-middle (MitM) cyberattacks.
Relying on deep learning convolutional neural networks (CNNs), the new cyber-physical system is meant to reduce the vulnerabilities of the robot operating system (ROS), which is used in both civilian and military robots.
Tested on a US Army GVT-BOT ground vehicle, the algorithm demonstrated a 99% accuracy, Fendy Santoso of Charles Sturt University and Anthony Finn of the University of South Australia (UniSA) note in their research paper (PDF).
The cyber-intrusion detection framework primarily focuses on detecting MitM attacks, but vulnerabilities in ROS make it prone to breaches, hijacking, denial-of-service (DoS), and other types of cyberattacks, the academics say.
These robots, the academics point out, are highly networked, because their different components, including sensors, actuators, and controllers rely on cloud services to transfer information and communicate.
“Robotic systems can be compromised at multiple different levels, namely, at the system, sub-system, component, or sub-component levels. Preventing these attacks is by no means trivial, especially for sophisticated, complex, and modern robots, which can work even under a fault-tolerant mode, blurring the line between normal operations and fault conditions,” the researchers note.
The MitM-detection algorithm was tested on ground robots that were connected to two separate computers over a Wi-Fi network. Once the cyberattack occurred, the robot became unresponsive, because the guidance signal it was supposed to receive was overwritten with unintended traffic data.
“As such, from the systems and control point of view, the robot was made blind with respect to the legitimate reference signal. This way, the attacker can also inject false data regarding the command signal to compromise the intended trajectory of the system,” the researchers note.
The collected data was used to train the cyber-intrusion system both under legitimate and cyberattack conditions, which ultimately yielded not only high accuracy, but also higher performance compared to other detection techniques in use, the academics claim.
The researchers are considering testing their algorithm on different robotic platforms, including unmanned aerial vehicles.
“Under the umbrella of deep learning (supervised and unsupervised) systems, we are also keen to study the relative merits of our CNN intrusion detection algorithm with respect to similar detection techniques such as using evolving type-2 fuzzy systems, that can accommodate the footprint-of-uncertainties,” the researchers say.
Related: Researchers Extract Sounds From Still Images on Smartphone Cameras
Related: New Wi-Fi Attack Allows Traffic Interception, Security Bypass
Related: ICS Vulnerabilities Chained for Deep Lateral Movement and Physical Damage
READ MORE HERE