ChatGPT Highlights a Flaw in the Educational System
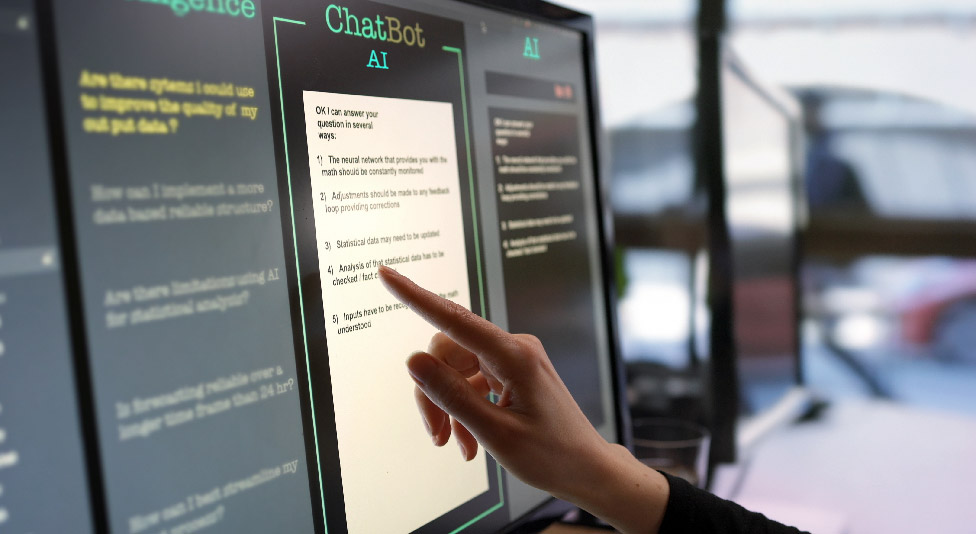
I recently participated in a conversation about artificial intelligence, specifically ChatGPT and its kin, with a group of educators in South Africa. They were concerned that the software would help students cheat.
We discussed two possible alternatives to ChatGPT: First, teachers could require that students submit handwritten homework. This would force students to at least read the material once before submitting it; Second, teachers could grade the paper submissions no higher than 89 percent (or a “B”), but that to get an “A,” the student would have to stand in front of the class and verbally discuss the material, their research, their conclusion, and answer any questions the teacher or other classmates might ask. (With that verbal defense of the ideas, the teacher might even waive the requirement for paper submission at all!)
The fundamental problem is that the grading system depends on homework. If education aims to teach an individual both a) a body of knowledge and b) the techniques of reasoning with that knowledge, then the metrics proving that achievement is misaligned.
One of the most quoted management scientists is Fredrick W. Taylor. He is most known for saying, “If you can’t measure it, you can’t manage it.” Interestingly, he never said that – which is fortunate because it is entirely wrong. People always manage things without metrics – from driving a car to raising children. He said: “If you measure it, you’ll manage it” – and he intended that as a warning. Whenever you adopt a metric, you will adjust your assessment of the underlying process in terms of your chosen metric. His warning is to be very careful about which metrics you choose.
Sometime in the past forty years, we decided that the purpose of education is to do well on tests. Unfortunately, that is also wrong. The purpose of education is to teach people to gather evidence and to think clearly about it. Students should learn how to judge various forms of evidence. They should understand rhetorical techniques (in the classical sense – how to render ideas clearly). They should be aware of common errors in thinking – the cognitive pitfalls we all fall into when rushed or distracted and logical fallacies which rob our arguments of their validity.
Large Language Models (LLMs) aggregate vast troves of text. Those data sources are not curated, so LLMs reflect the biases, logical limitations, and cognitive distortions in so much of what’s online. We are all familiar with early chatbots that were easily corrupted – the Microsoft chatbot Tay was perverted into being a racist resonator. (See “Twitter taught Microsoft’s AI Chatbot to be a Racist A**hole in Less than a Day” from The Verge, March 24, 2016, at https://www.theverge.com/2016/3/24/11297050/tay-microsoft-chatbot-racist accessed Aug 2023.)
LLMs do not think. They scan as much material as possible, then build a set of probabilities about which word is most likely to follow another word. If the word “pterodactyl” occurs in a text, then the next most likely word might be “soaring,” and “flying” might be in second place. If ChatGPT gets the word “pterodactyl” as input, it will put “soaring” next to it. This may look plausible to a person reading the output, but it cannot be correct. Correctness implies some kind of comprehension and judgment. ChatGPT does neither. It merely arranges words based on their statistical likelihood in the LLM’s database. We are now learning that LLMs that ingest computer-generated content become even more skewed – augmenting the likelihood of one word following another by rescanning the previous output. Over time, LLMs fed AI-generated content will drift farther and farther from actual human writing. The oft-mentioned hallucinations that LLMs generate will become more common as the distillation and amplification of the more likely subset of words leads to a contracted pool of possible machine-generated responses. Eventually – if we are not able to prevent LLMs from ingesting already-processed content – the output of ChatGPT will become more and more constrained, which, taken to the extreme, will yield one plot, one answer, one painting, and one outcome regardless of the specific input. Long before then, people will have abandoned LLM-based efforts for any activity that requires creativity.
Where can LLMs help? By sorting through bounded sets of information. That means an LLM trained on protein sequences could rapidly develop a most likely model for a protein that could attack a particular disease or interrupt an allergic reaction. In that case, the issue isn’t seeking creativity but rapidly scanning a set of nearly identical data overreactions to find the few that stand out enough to make a difference. A human doing this kind of work would quickly grow bored and likely make errors. LLMs can help science move quickly through vast quantities of data in closed domains. But when looking at an unbounded domain (art, poetry, fiction, movies, music, and the like), LLMs can only build average content, filling in the space between works. Artists seek to reach beyond the space their prior work defined.
The core problem with LLMs may be unsolvable. At this point, various organizations are exploring ways to tag AI-generated content (written and graphic) so humans can spend a moment assessing the accuracy and validity of the material. Of course, message digests can be corrupted and watermarks forged. A bad actor might maliciously tag authentic content as AI-generated. Recent developments include malicious ChatGPT variants designed to create BEC and phishing email content,
Students will always look for a shortcut, and that habit is difficult to overcome. In business, it will also be tempting for bureaucrats to use tools to simplify their tasks. How will your firm incorporate LLMs safely into your business processes? Organizations should consider how they will audit their internal procedures to ensure that LLM outputs are incorporated appropriately into communications. Imagine the potential for harm if some publicly traded company was found to have used an LLM to develop its annual financial report!
What do you think? Let me know in the comments below, or contact me @wjmalik@noc.social
Read More HERE