Securing AI: What You Should Know
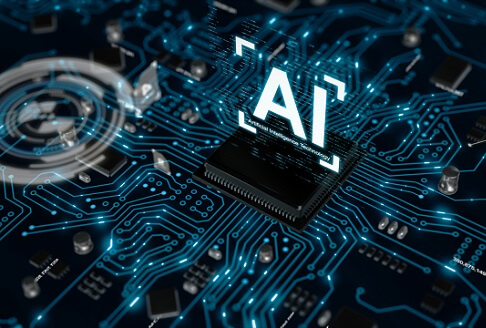
Machine-learning tools have been a part of standard business and IT workflows for years, but the unfolding generative AI revolution is driving a rapid increase in both adoption and awareness of these tools. While AI offers efficiency benefits across various industries, these powerful emerging tools require special security considerations.
How is Securing AI Different?
The current AI revolution may be new, but security teams at Google and elsewhere have worked on AI security for many years, if not decades. In many ways, fundamental principles for securing AI tools are the same as general cybersecurity best practices. The need to manage access and protect data through foundational techniques like encryption and strong identity doesn’t change just because AI is involved.
One area where securing AI is different is in the aspects of data security. AI tools are powered — and, ultimately, programmed — by data, making them vulnerable to new attacks, such as training data poisoning. Malicious actors who can feed the AI tool flawed data (or corrupt legitimate training data) can potentially damage or outright break it in a way that is more complex than what is seen with traditional systems. And if the tool is actively “learning” so its output changes based on input over time, organizations must secure it against a drift away from its original intended function.
With a traditional (non-AI) large enterprise system, what you get out of it is what you put into it. You won’t see a malicious output without a malicious input. But as Google CISO Phil Venables said in a recent podcast, “To implement [an] AI system, you’ve got to think about input and output management.”
The complexity of AI systems and their dynamic nature makes them harder to secure than traditional systems. Care must be taken both at the input stage, to monitor what is going into the AI system, and at the output stage, to ensure outputs are correct and trustworthy.
Implementing a Secure AI Framework
Protecting the AI systems and anticipating new threats are top priorities to ensure AI systems behave as intended. Google’s Secure AI Framework (SAIF) and its Securing AI: Similar or Different? report are good places to start, providing an overview of how to think about and address the particular security challenges and new vulnerabilities related to developing AI.
SAIF starts by establishing a clear understanding of what AI tools your organization will use and what specific business issue they will address. Defining this upfront is crucial, as it will allow you to understand who in your organization will be involved and what data the tool will need to access (which will help with the strict data governance and content safety practices necessary to secure AI). It’s also a good idea to communicate appropriate use cases and limitations of AI across your organization; this policy can help guard against unofficial “shadow IT” uses of AI tools.
After clearly identifying the tool types and the use case, your organization should assemble a team to manage and monitor the AI tool. That team should include your IT and security teams but also involve your risk management team and legal department, as well as considering privacy and ethical concerns.
Once you have the team identified, it’s time to begin training. To properly secure AI in your organization, you need to start with a primer that helps everyone understand what the tool is, what it can do, and where things can go wrong. When a tool gets into the hands of employees who aren’t trained in the capabilities and shortcomings of AI, it significantly increases the risk of a problematic incident.
After taking these preliminary steps, you’ve laid the foundation for securing AI in your organization. There are six core elements of Google’s SAIF that you should implement, starting with secure-by-default foundations and progressing on to creating effective correction and feedback cycles using red teaming.
Another essential element of securing AI is keeping humans in the loop as much as possible, while also recognizing that manual review of AI tools could be better. Training is vital as you progress with using AI in your organization — training and retraining, not of the tools themselves, but of your teams. When AI moves beyond what the actual humans in your organization understand and can double-check, the risk of a problem rapidly increases.
AI security is evolving quickly, and it’s vital for those working in the field to remain vigilant. It’s crucial to identify potential novel threats and develop countermeasures to prevent or mitigate them so that AI can continue to help enterprises and individuals around the world.
Read more Partner Perspectives from Google Cloud
Read More HERE